Emergent neural networks to redesign clinical testing of medicines and learning from real world evidence
Real World Data (RWD) and Real-World Evidence (RWE) are playing an increasing role in healthcare decisions to support innovative use of Electronic Health Records and other digital sources to help patients and physicians with experimental and existing treatments [R1]. For example: phase 3 clinical trials evaluating new therapies and vaccines are among the most complex experiments performed in medicine. Around 50% of phase 3 trials fail and, in some cases, lead to adverse events [R2]. This high failure rate also counteracts patients consenting to treatments by experimental drugs as a last resort. The United States Food and Drug Administration (FDA) states that another significant challenge is the difficulty of predicting clinical results in a wider patient base in the real world vs. controlled randomized trials [R1]. In collaboration and with guidance from the United States FDA, my research lab invents explainable AI and machine learning systems that learn from diverse and inclusive datasets for ethical clinical use and testing of experimental medicines [R3].
- For example, I have led research studies that have described novel and non-trivial reward functions for self-learning Reinforcement Learning (RL) algorithms for dose de-escalation studies during clinical trials to alleviate chemotherapy toxicity. These algorithms learn reward contribution from physician actions and patient states/health without future survival/outcomes information to solve fundamental problems in clinical development of medicines [1].
- I served as the sole principal investigator on the Memorandum of Understanding (MOU) ‘Health 0.0’ between MIT and the United States FDA signed to engender AI and ML research for computational medicine and clinical development. Research activities led by me as the Principal Investigator under this MOU focus on three key themes for development of next-generation medicines by adoption of digital evidence generated by AI and ML: (1) validation and modernizing the clinical trials process, (2) strategies for rational use of AI- and ML-driven learning from real-world data and evidence and, (3) regulatory framework to improve health outcomes for patients and oversight for integration, explanation, and de-risking of AI/ML digital analytics in medical care to patients [2, R3].
- In collaboration with regulatory agencies and clinical partners, I am initiating a regulatory path for AI and ML software as a medical device and digital medicines developed in my laboratory from this research [2, 4, 6]. Research from my lab classifies, predicts, and enriches novel digital endpoints to benefit patient health, eliminate adverse events, and improve outcomes. This work has significant impact on the ethical decisions facing patients and their families, and regulatory decisions for US FDA and European Medical Agency [2, R1].
- In another effort, I am leveraging the use of observational RWD and RWE to supplement randomized data to train neural networks cognizant of causal inference [3, 5].
Peer-Reviewed Publications
-
Reinforcement learning with action-derived rewards for chemotherapy and clinical trial dosing regimen selection. 2018. [Abstract] [Full Paper]
Gregory Yauney, Shah P*
(*Senior author supervising research)
Proceedings of the 3rd Machine Learning for Healthcare Conference. PMLR 85:161-226
-
Artificial intelligence and machine learning in clinical development: a translational perspective. 2019. [Abstract] [Full Paper]
Pratik Shah* et al.
(*Corresponding author)
Nature Digital Medicine. DOI: 10.1038/s41746-019-0148-3
-
Artificial Intelligence for clinical trial design. 2019. [Abstract] [Full Paper]
Stefan Harrer, Shah P, Antony P, Hu J
Trends in Pharmacological Sciences. PMID: 31326235
-
Improving cancer diagnosis and care: clinical application of computational methods in precision oncology. 2019. [Abstract] [Full Paper]
Proceedings of a Workshop, National Academies of Sciences, Engineering, and Medicine. Washington, DC: The National Academies Press. PMID: 31386317
-
Artificial neural networks detect and classify hematologic malignancies or solid tumors using electronic medical records prior to clinical diagnosis. 2020.
Yujia Zhou, Barnes C, Magoc T, Lipori G, Shah P, Cogle CR
Published at 61st American Society of Hematology Annual Meeting, Orlando, FL
-
Data science in clinical pharmacology and drug development for improving health outcomes in patients. 2020. [Abstract] [Full Paper]
Pratik Shah, Peck R, Vamvakas S, van der Graaf, Piet H
Clinical Pharmacology and Therapeutics. PMID: 32202650
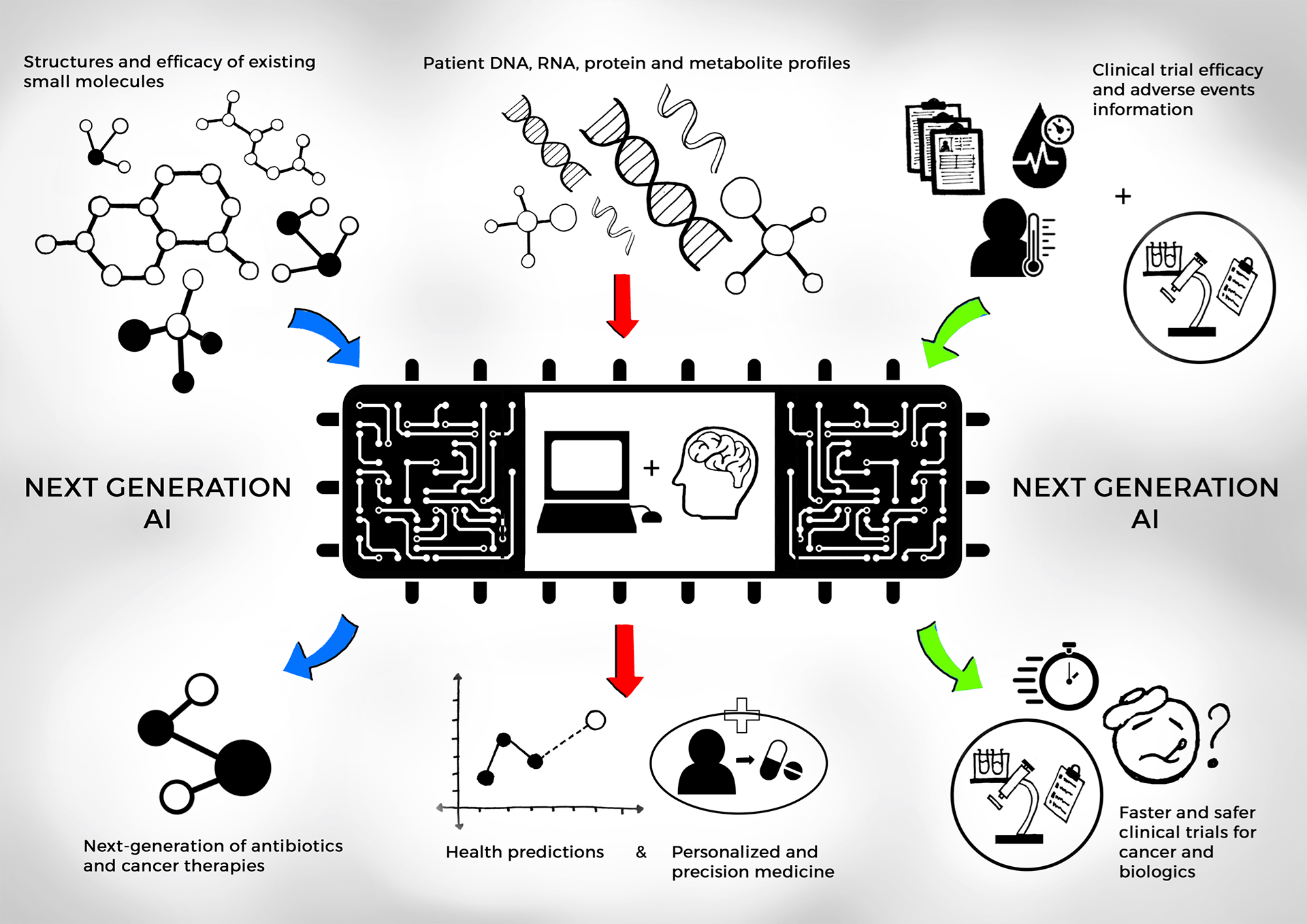
Resources
- R1 - Framework for FDA’s Real-World Evidence Program [PDF]
- R2 - 22 Case Studies Where Phase 2 and Phase 3 Trials had Divergent Results [PDF]
- R3 - FDA MOU [Link]
Select Talks
- 2021 - Regulatory landscape and societal impact of clinical decision making and therapeutic development driven by AI technologies
- 2020 - Electronic health records and clinical decision making for real world evidence
- 2019 - Machine learning systems and discovery of actionable biomarkers for clinical development
- 2019 - Career paths at the intersection of clinical medicine and emerging technologies
- 2019 - Machine learning driven computational medicine for oncology care
- 2019 - Artificial intelligence and machine learning for regulatory science and clinical development applications
- 2019 - Machine learning and computational medicine for clinical development, patients and regulators
- 2018 - Data regulation and privacy for clinical trials
- 2018 - Digital clinical trials for oncology patients with novel machine learning and AI architectures
- 2018 - Future of digital medicine and clinical trials with novel machine learning and AI architectures
- 2017 - Artificial Intelligence in Clinical Development to Improve Public Health
Press
- 2019 - Faster Drug Approvals Possible as AI Speeds FDA Reviews
- 2019 - Pratik Shah invited to join editorial leadership of The American Society for Clinical Pharmacology & Therapeutics flagship journal Clinical Pharmacology & Therapeutics
- 2018 - Artificial intelligence model “learns” from patient data to make cancer treatment less toxic